Home | Introduction | Metadata | Application | Further reading | Landscape of resources |
Application
- Clinical Trial Registries
- Background
- FAIR assessment of clinical trial registries
- Clinical Real World Data
- Other registries and efforts
- Quality and Governance of Clinical data
- Quality of clinical data and metadata
- Clinical data governance at the study level
- Infrastructure, training and culture for clinical FAIR data
- Infrastructure
- Education and training
- Data-centric culture and the FAIR mindset
Clinical Trial Registries
Background
FAIR implementation is one of the fundamental enablers for secondary reuse of clinical data, thereby realising much greater value from our data assets. FAIR clinical data and metadata will be findable (discoverable) by both machines and humans, any access restrictions will be determined by open protocol standards and supported by highly expressive FAIR vocabulary or ontology standards, including formats. These attributes of Findability, Accessibility and Interoperability together support Reusability which includes the attributes of data usage, provenance and community standards as illustrated in Figure 10.
Figure 10: FAIR as an enabler for secondary use of clinical data. This is supported by clinical data being sufficiently Findable, Accessible, Interoperable and Reusable for action by machines and humans.
Registries for clinical interventional and observational studies are important resources for supporting the secondary use of clinical data. Examples include Clinical trial registries such as those provided for the FDA by the NIH (https://clinicaltrials.gov), the EU (https://www.clinicaltrialsregister.eu/) and Cochrane (https://www.cochranelibrary.com/central) which are within the scope of this guide. For each study, they usually include a study description, the study protocol and summary data for the trial results.
In order to assess how FAIR these clinical registers are now, and what improvements could be made, breast cancer and Type 2 diabetes have been selected as use cases. These use cases are also representativeattractive because they are often found in the therapeutic portfolios of many pharmaceutical companies. Furthermore, there is much evidence in the literature for comorbidity between the two conditions see for instance Zhao et al. (2016), Yoon SJ et al. (2015) and Samuel SM et al. (2018).
FAIR assessment of clinical trial registries
ClinicalTrials.gov
The NIH funded registry, https://clinicaltrials.gov is the largest open clinical trials database which has been available online since the year 2000. In the web-based user interface, Basic text search (e.g. see query results for “Diabetes Mellitus, Type 2”) is provided along with the advanced search that provides additional search facets, e.g. eligibility criteria, locations or start and end date of a study.
The registry does offer an API for bulk download of the study methods (metadata) and results summary (data). The available actions and (meta)data elements available from the API corresponds to the advanced search interface and structured data submission.
For assessment of FAIR maturity we selected two examples, completed clinical trial study with results for each of 1) Diabetes Mellitus Type 2, NCT01020123 (URL or URL for beta) and 2) Female Breast Cancer, NCT00633464 (URL and URL for beta) as shown in Table 4. Note that the content of the beta website is identical to the current, so the assessment of FAIR maturity applies to both.
Table 4: Assessment of ClinicalTrials.gov using the simplified FAIR Maturity Indicators based on two example completed studies with results for the two selected disease conditions.
FAIR pillars | Simplified FAIR Maturity indicators (MIs) for data and metadata | Formal FAIR MIs | Priority | Clinicaltrial.gov | FAIR Score for study |
---|---|---|---|---|---|
Findable | Global Unique Persistent Resolvable identifiers (GUPRI) | F1, F2, F3 | Essential | GUPRI for study | 1 |
Uniform Resource Locator (URL) | F4 | Desirable | URL for study | 2 | |
Accessible | Open standard protocol for identifier resolution to support authentication and authorisation for access to restricted content | A1 | Essential | Unrestricted access to method and results summary (no patient data) | |
Metadata has a persistence policy for discoverability independent from the associated data | A2 | Desirable | Legal obligation for retention of the registry | 1 | |
Interoperable | FAIR vocabularies or ontologies with external links and language for knowledge representation | I1, I2, I3 | Desirable | Structured but no linked terminology nor knowledge representation | 1 |
Reusable | Attributes for consent for data usage (licence and owner), provenance (e.g. PROV ontology) and use of relevant clinical standards (e.g. CDISC, OHDSI, FHIR etc.) | R1, R2, R3 | Essential | Study submitter and registry policy and legislation. No provenance or clinical standards. | 1 |
TOTAL | 2 is fully satisfied, 1 is partial, 0 not satisfied | Max possible: 10 | 6 |
Findable The study identifiers, NCT01020123 and NCT00633464 are global, unique, persistent and resolvable by machine (GUPRI) which has been incorporated into the Uniform Resource Locator (URL: https://clinicaltrials.gov/ct2/show/study/NCT01020123) which is discoverable by Google search. This satisfies the four FAIR maturity indicators for Findability (F1, F2, F3 and F4) at the study level (see table 4). The same studies can be retrieved as xml which corresponds to the tabular view in html to expose the structured metadata in a machine-readable format.
Accessible The results summary for this clinical trials registry is at the study rather than patient level so access is unrestricted which does not require authentication or authorisation for viewing (see table 4). The persistence policy for the results summary data and study details metadata is likely to be long term although this is not stated explicitly on the history and policies page.
Interoperable Even though the metadata for the study description is structured (tabular view or xml), it consists of much free text and almost no identifiers for vocabulary or ontology terms can be found. In this regard, the FAIR interoperability of the clinicaltrial.gov registry could be much improved. Further opportunities to make this registry more FAIR include: 1) JSON Linked Data API instead of plain JSON, 2) Adoption of the openAPI/SmartAPI (link) specifications for FAIR services and 3) Convert XML based data schema to an ontology powered schemaConvert data schema (XSD) to an Ontology., Tconvert term lists could be converted to SKOS-CVs, align/cross-map to dominant FAIRsharing terminologies. Adoption of the CDISC vocabulary is also likely to improve this clinical trials registry (see table 4).
Reusable The use of data is described at https://clinicaltrials.gov/ct2/about-site/terms-conditions which is protected by international copyright outside the USA or some third parties. Guidance is given to study record managers on submission to ClinicalTrials.gov using the Protocol Registration and Results System (PRS). This provides the only mechanism for expression of provenance and maintaining the expected standards, policies and legal obligations for this community. This mechanism would benefit from use of the provenance ontology, PROV and by adoption of the major clinical standard, CDISC.
EU Clinical Trials Register
The EU Clinical Trials Register (EU CTR) comprises of method protocols and summary results information for interventional clinical trials similar to Clinical Trials.gov.
Basic text search for “Diabetes Mellitus, Type 2” yields more than 1500 results (see figure YY), a summary of the study level data for every search hit is displayed, including e.g. identifiers for the trial, the sponsor name, the title of the trial, Mmedical condition, Ddisease, Ppopulation age. Additional metadata and summary data for the trial results is organised in the following categories, similar to Clinical Trials.gov:
- Trial Information
- Subject disposition
- Baseline characteristics
- End points
- Adverse events
- More information
The graphical user interface also offers more advanced search besides text search with logical operators, to enable filter of the results by facets such as Country, Age Range, Trial Status, Trial Phase or Gender.
EU CTR allows the user to download trial results after successful search as a plain text rather structured file which mirrors the free text pdf format for the results, see figure ZZ. However CTR does not offer API access for bulk download.
For assessment of FAIR maturity we compared the same two examples from completed clinical trial studies with results for each of 1) Diabetes Mellitus Type 2, 2009-012612-41 (URL) [=NCT01020123] and 2) Female Breast Cancer, 2007-005209-23 (URL) [=NCT00633464] as shown in table 5.
Table 5: Assessment of EU CTR using the simplified FAIR Maturity Indicators based on two example completed studies with results for the two selected disease conditions.
FAIR pillars | Simplified FAIR Maturity indicators (MIs) for data and metadata | Formal FAIR MIs | Priority | EU CTR | FAIR Score for study |
---|---|---|---|---|---|
Findable | Global Unique Persistent Resolvable identifiers (GUPRI) | F1, F2, F3 | Essential | Identifier for study | 1 |
Uniform Resource Locator (URL | F4 | Desirable | URL for study (via query) | 1 | |
Accessible | Open standard protocol for identifier resolution to support authentication and authorisation for access to restricted content | A1 | Essential | Unrestricted access to method and results summary (no patient data) | |
Metadata has a persistence policy for discoverability independent from the associated data | A2 | Desirable | Legal obligation for retention of the registry | 1 | |
Interoperable | FAIR vocabularies or ontologies with external links and language for knowledge representation | I1, I2, I3 | Desirable | Free text, no structure (pdf dump) | 0 |
Reusable | Attributes for consent for data usage (licence and owner), provenance (e.g. PROV ontology) and use of relevant clinical standards (e.g. CDISC, OHDSI, FHIR etc.) | R1, R2, R3 | Essential | Study submitter and registry policy and legislation. No provenance or clinical standards. | 1 |
TOTAL | 2 is fully satisfied, 1 is partial, 0 not satisfied | Max possible: 10 | 4 |
Findability: The study identifiers, 2009-012612-41 (=NCT01020123) and 2007-005209-23 (=NCT00633464) are unique and resolvable by machine but the globality and persistence of these identifiers is open to question. These identifiers have been incorporated into the URL (e.g. https://www.clinicaltrialsregister.eu/ctr-search/search?query=2009-012612-41) which can be found using google search. This partially satisfies the four FAIR maturity indicators for Findability (F1, F2, F3 and F4) at the study level (see table 5).
Accessibility: The results summary for this clinical trials registry is at the study rather than patient level so access is unrestricted which does not require authentication or authorisation for viewing (see table 5). The persistence policy for the results summary data and study details metadata is likely to be long term although this is not stated explicitly. It is notable that CT registry limits user access to a graphical user interface, so there is no programmatic access (i.e. no API) for batch download, unlike http://clinicaltrials.gov .
Interoperability: The study methods and results data and metadata are only available in an unstructured format as plain text or as pdf. The content is identical to that found in ClinicalTrials.gov for the two example trial records. This means there are almost no identifiers for vocabulary or ontology terms. Clearly, the FAIR interoperability of the EU CTR is a massive opportunity for improvement.
Reusable: The use of data is described in the “about the EU clinical trials register” section which is protected by legal rights described at this page. EU CTR would benefit from use of a provenance ontology, such as PROV and by adoption of the major clinical standard, such as CDISC.
Cochrane Library Central for Controlled Trials
The Cochrane Library Central for Controlled Trials (CENTRAL) is a registry of controlled trials, owned by the publisher John Wiley & Sons Ltd. Most CENTRAL records are taken from bibliographic databases (mainly PubMed and Embase.com) and they are also derived from other sources, including CINAHL, ClinicalTrials.gov and the WHO’s International Clinical Trials Registry Platform. Therefore, CENTRAL is best seen as a secondary registry for clinical trials (about CENTRAL).
Table 6: Assessment of CENTRAL using the simplified FAIR Maturity Indicators based on two example completed studies with results for the two selected disease conditions.
FAIR pillars | Simplified FAIR Maturity indicators (MIs) for data and metadata | Formal FAIR MIs | Priority | Cochrane Central Register of Controlled Trials | FAIR Score for study |
---|---|---|---|---|---|
Findable | Global Unique Persistent Resolvable identifiers (GUPRI) | F1, F2, F3 | Essential | Local identifier (not GUPRI) | 0 |
Uniform Resource Locator (URL | F4 | Desirable | Not discoverable by Google search | 0 | |
Accessible | Open standard protocol for identifier resolution to support authentication and authorisation for access to restricted content | A1 | Essential | Open access to abstract, info and related content | |
Metadata has a persistence policy for discoverability independent from the associated data | A2 | Desirable | Commercial resource subject to business decisions | 1 | |
Interoperable | FAIR vocabularies or ontologies with external links and language for knowledge representation | I1, I2, I3 | Desirable | A few structured fields including a link to the primary source, ClinicalTrial.gov record | 1 |
Reusable | Attributes for consent for data usage (licence and owner), provenance (e.g. PROV ontology) and use of relevant clinical standards (e.g. CDISC, OHDSI, FHIR etc.) | R1, R2, R3 | Essential | Open access policy for usage. Provenance and clinical standards depend on the primary source. | 1 |
TOTAL | 2 is fully satisfied, 1 is partial, 0 not satisfied | Max possible: 10 | 3 |
Findability The study identifiers, CN-01526935 (=NCT01020123), URL and CN-01517484 (=NCT00633464), URL are local identifiers rather than GUPRI. These identifiers have been incorporated into the URL (e.g. https://www.cochranelibrary.com/central/doi/10.1002/central/CN-01526935/full) but it is not discoverable using Google search. This does not satisfy the FAIR maturity indicators for Findability (F1, F2, F3 and F4) at the study level (see Table 6)
Accessibility The registry record is limited to an abstract, info keywords and related content at the study level, based on the primary source, ClinicalTrials.gov. So access to the content does not require authentication or authorisation for viewing . The persistence policy for the results summary data and study details metadata is not stated explicitly but likely to depend on the primary source. Furthermore, persistence will be subject to the business decisions of a commercial publisher. This only partially satisfies the FAIR maturity indicators for accessibility (Table 6).
Interoperability The registry content is available as plain text and in numerous structured formats, much like bibliography registers such as PubMed. The content is a condensed derivative from the primary source, ClinicalTrials.gov for the two example trial records. This means there are almost no identifiers for vocabulary or ontology terms which would improve the FAIR interoperability, thereby adding value to CENTRAL.
Reusable Cochrane has an open access policy for data usage. As a secondary registry, CENTRAL is likely to be dependent on its primary sources for provenance and clinical standards.
Clinical Real World Data
Definitions of clinical Real World Data and Real World Evidence
Real-world data (RWD) is a general term for data that results from the effects of health interventions (such as benefits, risks or resource use) that are not collected in the context of double-blind, randomised, controlled trials (RCTs).
While definitions may vary, RWD tends to be structured, having ‘data models’ with data residing in a fixed field, for example in databases and spreadsheets. RWD can be collected both prospectively and retrospectively from observations of routine clinical practice. Data collected may include, but are not limited to, clinical and economic outcomes, patient-reported outcomes and health-related quality of life.
The analysis of RWD is undertaken to derive Real World Evidence (RWE) which underpins the effectiveness of existing therapies to help plan studies of new medicines. In addition, the RWE of safety outcomes is frequently required by regulatory agencies after marketing authorisation.
GetReal RWE Navigator
The RWE Navigator from the IMI GetReal project includes sources of RWD which lists five types of RWD sources, as summarised in the table below, which also considers the structure expected for the type of RWD source.
Table 7: Types of RWD sources
RWD source | Short description | Structure of RWD |
---|---|---|
Patient Registries | Patient organisations use these to prospectively collect, analyse, and disseminate observational data on a group of patients with specific characteristics in common. They are cohort studies and data is collected electronically, usually in databases | The database schema provides the structure. |
Healthcare Databases with a Focus on Electronic Health Records | Systematic collections of electronic health records (EHRs), into which healthcare providers enter routine clinical and laboratory data during usual practice. Healthcare databases can be used in ‘real-world’ (observational) studies to assess the benefits and risks, as well as the relative effectiveness, of different medical treatments. | The database schema provides the structure. |
Pharmacy and Health Insurance Databases | Set up by pharmacists or health insurers for billing and other healthcare administration and management, such as monitoring of healthcare service use. Data collected in these systems can also be used in medical research to assess the effectiveness of healthcare interventions in ’real world’ observational studies. | The database schema provides the structure. |
Social Media | Internet-based platforms that enable users to create and share content or to participate in social networking. They can provide patient perspectives on health topics such as adverse events, reasons for changing treatments and non-adherence, and quality of life. | Mostly free text with no structure. |
Patient-Powered Research Networks | Online platforms run by patients to collect and organise health and clinical data. | Mostly free text with no structure. |
The registries and databases for RWD are highly structured whereas these are mostly free text for social media and patient-powered research networks. A formidable response to meet this challenge for the rapid proliferation of rare disease registries was the formation of the European Joint Programme on Rare Diseases which includes numerous resources to support FAIRification of RWD. As a result Kodra et al (2018) published the paper entitled “Recommendations for Improving the Quality of Rare Disease Registries”.
ClinicalTrials.gov
Besides interventional clinical trials, ClinicalTrials.gov also includes over 3,009 available and completed observational studies with results which can be seen as a more organised form of RWD. ClinicalTrials.gov defines observational studies as “a type of clinical study in which participants are identified as belonging to study groups and are assessed for biomedical or health outcomes. Participants may receive diagnostic, therapeutic, or other types of interventions, but the investigator does not assign participants to a specific interventions/treatment.” - as defined here.
As of October 2022, ClinicalTrials.gov includes available and completed observational studies with results for the selected use cases, Type 2 diabetes (N = 81) and breast cancer with female filter (N = 65). The assessment of FAIR maturity described previously applies to the observational studies too. ClinicalTrials.gov also has three additional observational studies originating from patient registries for Type 2 diabetes and none for breast cancer.
Global Alliance for Genomics and Healthcare
Global Alliance for Genomics and Healthcare (GA4GH) is a source of standards and tools for real world genomic and health-related data with over 600 organisations. These are mostly academic institutions, SMEs and a few major Pharmaceutical companies. GA4GH lists a collection of Driver Projects which are real-world genomic data initiatives.
Below are the current GA6GH driver projects (n=24 on 1st June 2022):
- All of Us Research Program
- Australian Genomics
- Autism Sharing Initiative
- BRCA Challenge
- Canadian Distributed Infrastructure for Genomics (CanDIG)
- Clinical Genome Resource (ClinGen)
- ELIXIR Beacon
- ELIXIR Cloud and AAI
- ENA / EVA / EGA
- EpiShare
- EUCANCan
- European Joint Programme on Rare Disease
- GEnome Medical alliance Japan (GEM Japan)
- Genomics England
- Human Cell Atlas
- Human Heredity and Health in Africa (H3Africa)
- ICGC-ARGO
- Matchmaker Exchange
- Monarch Initiative
- National Cancer Institute Cancer Research Data Commons (NCI GDC)
- National Cancer Institute Genomic Data Commons (NCI GDC)
- Swiss Personalized Health Network (SPHN)
- Trans-Omics for Precision Medicine (TOPMed)
- Variant Interpretation for Cancer Consortium (VICC)
The Driver Projects have helped GA4GH to guide their development efforts, pilot tools and advocate, mandate, implement and use relevant frameworks and standards, such as phenopackets and GA4GH news. The documentation for Phenopackets schema includes a link to the FHIR Implementation Guide, Phenopacket building blocks, a list of 10 recommended ontologies and three complete examples for Rare Disease, Cancer and a COVID-19 case report.
How FAIR are these sources of real world clinical data at the study level?
The Genomic Data Toolkit collection of GA4GH adopts and shares open standards for genomic data sharing and is made available through Github repositories for code, example files and data. It also provides recommendations for various API’s, data file formats, BED specifications, Data Use Ontologies (DUO) and many more resources.
GA4GH driver projects are discoverable via the general web link and Google search. The data for each GA4GH driver project are available through controlled data access procedures, such as used by dbGaP, which supports look up, for example the specific TOPMed Whole Genome Sequencing study. These studies will release data according to patient consent and scope as described in each individual study, in which patients have expressed their wishes for which projects the data will and will not be allowed to be used.
Data in the GA4GH Driver project studies are FAIRified to varying degrees for each initiative. For example, TOPMed (NHLBI Trans-Omics for Precision Medicine) has implemented FAIR for several of its participating studies. The FAIR maturity assessments have been undertaken using the FAIRshake tool which finds that most of these studies lack a study identifier which is global, unique, persistent and resolvable by machines. This is an obvious opportunity for improvement. A further example, which we have undertaken a simplified FAIR assessment for, is the Australian Genomics data collection, as described next.
Table 8: Assessment of the Australian Genomics Data collection using the simplified FAIR Maturity Indicators based on the data catalogue and web site. For the collection.
FAIR pillars | Simplified FAIR Maturity indicators (MIs) for data and metadata | Formal FAIR MIs | Priority | Australian Genomics Data collection | FAIR Score for study |
---|---|---|---|---|---|
Findable | Global Unique Persistent Resolvable identifiers (GUPRI) | F1, F2, F3 | Essential | No study identifiers evident in data catalogue | 0 |
Uniform Resource Locator (URL) | F4 | Desirable | URL for collection website | 1 | |
Accessible | Open standard protocol for identifier resolution to support authentication and authorisation for access to restricted content | A1 | Essential | Unrestricted access to the data catalogue (restricted access to study or patient data). No open standard protocol. | 1 |
Metadata has a persistence policy for discoverability independent from the associated data | A2 | Desirable | No persistence policy evident | 0 | |
Interoperable | FAIR vocabularies or ontologies with external links and language for knowledge representation | I1, I2, I3 | Desirable | Structured data fields with some linked terminology and ontology mapping | 1 |
Reusable | Attributes for consent for data usage (licence and owner), provenance (e.g. PROV ontology) and use of relevant clinical standards (e.g. CDISC, OHDSI, FHIR etc.) | R1, R2, R3 | Essential | Agreements and policy to apply for access. Vocabularies, ontologies and FHIR clinical standards. Limited provenance. | 1 |
TOTAL | 2 is fully satisfied, 1 is partial, 0 not satisfied | Max possible: 10 | 4 |
Findability: The Australian Genomics data web site is findable with Google search. Data capture and standardisation is addressed on a web page, which makes no mention of the FAIR data maturity. There is a catalogue for all the datasets which has no identifiers and takes the form of a downloadable Excel workbook with a sheet for each dataset. An obvious opportunity for improvement is to assign each dataset, which is an observational study, an identifier, ideally GUPRI.
Accessibility: A typical example of FAIR maturity for accessibility is the Australian Genomics data where access for secondary reuse can be applied for using application forms available for download for each disease area. A catalogue snapshot as an Excel file. No open standard access protocol is evident which is a clear opportunity for improvement.
Interoperability: For the Australian Genomics data, the data catalogue sheets indicate that many of the datasets have data field structure and include or map to ontologies such as HPO and SNOMED. Although this is encouraging, more in depth assessment of interoperability requires application for access to a particular dataset via one of the application forms, which is beyond the scope of this guide.
Reusability: For the Australian Genomics data, the policy for data access and sharing for secondary use, data access and sharing agreement and data breach policy are all available on a web page.
Challenges and a relevant initiatives for RWD and RWE
The example of Australian Genomics data illustrates the many challenges and opportunities to improve the FAIR maturity of these clinical genomic datasets for secondary reuse. We expect this finding will be all too common for clinical RWD, even at the study rather than patient level. A relevant paper from Cave et al. 2019 is entitled “Real‐World Data for Regulatory Decision Making: Challenges and Possible Solutions for Europe”.
The European Medicines Agency (EMA) and some national medicines agencies have been investing in access to RWD. Building on this experience, in 2021, the European Medicines Regulatory Network (EMRN) initiated plans to create an EU-wide distributed network of RWD named the Data Analytics and Real World Interrogation Network (DARWIN EU). Similarly, the US FDA has their Real Word Evidence Program which includes documentation for the RWE Program RWE Program Framework, published in 2018. Ideally, such multinational RWD initiatives should be guided by FAIR principles, rather than creating clinical data silos which are all too often lost to secondary reuse.
Other registries and efforts
We just want to briefly mention and link out to other relevant data registries and efforts, without going into further details or FAIR assessments:
- The Translational Data Catalog, a joint effort among IMI-FAIRplus, IMI-eTRIKS and ELIXIR-Luxembourg, tries to improve FAIRness for (IMI) datasets and encourage data sharing. It embeds schema.org and bioschemas, which is a lightweight but effective approach. The catalog distinguishes “project”, “studies” and “datasets” and plans to make all those searchable in the future (e.g. see search for “Type 2 diabetes” here).
- The International Clinical Trials Registry Platform (ICTRP) is another secondary trial register, created by the WHO, which makes data from multiple sources available. The search portal can be found at https://trialsearch.who.int/.
The European Medicines Agency published a list of metadata for Real World Data catalogues. This list contains metadata elements for describing real-world data (RWD) sources and observational studies.The chosen metadata will be included in a catalogue of data sources containing information about existing real-world databases (to replace the current ENCePP catalogue) and information about the studies performed on the data sources (to replace and enhance the current EU PAS Register). More information can be found at https://www.ema.europa.eu/en/documents/other/list-metadata-real-world-data-catalogues_en.pdf
Quality and Governance of Clinical data
Quality of clinical data and metadata
As discussed recently by Harrow et al. 2022, the FAIR data principles gain increasing popularity and acceptance. It is easy to assume that implementation of FAIR would be sufficient to drive the data management strategy in the life sciences. For example, comprehensive assessment using the FAIR metrics can result in valuable recommendations for improving the quality of rare disease registries. However, the FAIR principles only indirectly consider data quality at the level of provenance and meeting community standards for the whole data set. These are likely to be insufficient measures of data quality, especially in strictly governed environments, such as submissions of clinical trial data to the regulators by pharmaceutical companies, or electronic healthcare records (EHRs) kept by hospitals. Thus, it is not surprising that methods and dimensions for quality assessment have been developed for reuse of clinical data from EHRs.
Data quality assessment of electronic health records (EHRs)
Data Quality Assessment (DQA) of electronic healthcare records (EHRs) starts with analysis of relevant abstracts from the biomedical literature in PubMed to identify the dimensions of data quality and common methods to assess data quality, as described by Weiskopf and Weng. Five dimensions of data quality have been identified as: (i) completeness; (ii) correctness; (iii) concordance; (iv) plausibility; and (v) currency, which were mapped to seven methods for data quality assessment: (i) gold standard; (ii) data element agreement; (iii) element presence; (iv) data source agreement; (v) distribution comparison; (vi) validity check; and (vii) log review. The strongest evidence from this mapping was found between the dimensions of (i) completeness and (ii) correctness to the methods of (i) gold standard, (iii) element presence and (ii) data element agreement (Figure 11) .
Figure 11. Mapping between dimensions of data quality and data quality assessment methods. Dimensions are listed on the left and methods of assessment on the right, both in decreasing order of frequency from top to bottom. The weight of the edge connecting a dimension and method indicates the relative frequency of that combination. Reproduced, with permission, from Weiskopf 2013
Related work harmonised a greater number of data quality terms to design a more complex conceptual framework for defining whether EHR data are ‘fit’ for specific uses. [Ref]. This DQA framework comprises three broad categories: (i) conformance to specified standards or formats; (ii) completeness to evaluate data attribute frequency within a data set without reference to the data values; and (iii) plausibility with respect to a range or distribution of data values. Each of these categories include the following seven subcategories: (ia) value conformance; (ib) relational conformance; (ic) computational (calculation) conformance; (ii) completeness; (iiia) unique plausibility; (iiib) atemporal plausibility; and (iiic) temporal plausibility. All of these apply to metadata elements and data values, with the exception of computational conformance, which only applies to data values. Review of cardiac failure research study guidelines led to the identification of six categories of frequently used and clinically meaningful phenotypic data elements: (i) demographics; (ii) physical examination or baseline observation; (iii) diagnostic tests; (iv) patient medical history; (v) clinical diagnoses or presentation; and (vi) medications. These enabled the assembly of an inventory framework for the data elements, organised by the six categories of phenotype.[Ref] This is an example of DQA framework application to research studies from cardiac failure research.
Wider applicability of DQA to clinical data in addition to FAIR (FAIR+Q)
The DQA framework approach can be applied to other types of clinical data, such as those found in clinical trial registries, health claims databases, and health information exchanges. This approach can also be applied more broadly by compiling relevant harmonised terms (e.g., from existing vocabularies, ontologies, or natural language processing) as the starting point to identify the most relevant dimensions of data quality and methods for assessment, many of which are likely to be shared within the clinical domain.
FAIRification combined with quality assessment for clinical data
Although making clinical data FAIR is likely to release more value, this will probably be insufficient because the quality of data is only addressed indirectly, through unspecified provenance and community standards. Quality assessment is crucial for clinical trial and healthcare data for submission to regulators to demonstrate the efficacy and safety of a new treatment. Therefore, it is not surprising to find that assessment of quality for clinical data, especially in EHRs, has matured as described in the previous section. Here, we argue that it would have a greater impact to apply both the FAIR data metrics (maturity indicators) and data quality assessment (FAIR + Q) to clinical trial and healthcare data sets. The process of FAIR + Q assessment followed by enhancement of metadata and selection of quality data sets, guided iteratively by use cases, is likely to release maximum value from clinical data, while satisfying the rigour of regulatory submissions and vital decision-making of healthcare services.
Clinical data governance at the study level
What is data governance?
Figure 12: How Data Governance relates to FAIR Data Management in a typical large enterprise, such as a biopharmaceutical company
How does data governance relate to FAIR clinical data at the study level?
A number of recommendations for improving the quality of Rare Disease registries have been published in 2018 by Kodra and coauthors. They describe a framework for quality management of RD registries which includes establishment of a good governance system and construction of a suitable computing infrastructure which complies with the FAIR principles (see figure 1 in the review paper).
Five recommendations for governance of a Rare Disease registry are given: 1) Define clear objectives to inform the design of the registry database, 2) Identify and engage with the relevant stakeholders at an early stage, 3) Build the registry team who have clear roles and responsibilities in proportion to the registry size, ambitions and objectives, 4) Build a solid framework to ensure compliance with ethical and legal requirements and 5) Ensure the required budgets have been evaluated, so that the registry is well resourced for a predefined period.
Recommendations such as these should take account of registry scope; at the study level only protocol methods and summary results will be disclosed, rather than personal patient data. Therefore, for this FAIR4Clin guide, which is limited to the study level, we have described already how it is only relevant to consider FAIR implementation for the methods description of protocol (metadata) and results summary (data), submitted to the ClinicalTrials.org registry.
Infrastructure, training and culture for clinical FAIR data
Infrastructure
Effective FAIR data management requires sufficient investment in building a suitable infrastructure and support team to match the size and ambition of the business organisation. Numerous examples of such FAIR data enabled infrastructure implemented by major pharmaceutical companies have emerged in recent years.
The EDISON platform and FAIRification of clinical trial data at Roche
Roche has built the EDISON platform to enable prospective FAIRification of data at the point of entry to Roche, by harmonising, automating and integrating very heterogeneous and complex processes across multiple departments, building in data standards and quality checks for data models in clinical and non-clinical data. The EDISON platform is built as an ecosystem of self-contained micro services to ensure maximum performances, scalability and low maintenance. The current scope of EDISON is clinical non-CRF data but the platform is scalable and flexible to cover a large variety of data models, both clinical and non-clinical. More details about the EDISON platform can be found described as a use case in the Pistoia Alliance Toolkit: Prospective FAIRification of Data on the EDISON platform.
Roche launched a series of clinical use cases in 2017 with smaller data sets to answer specific scientific questions prioritised by a scientific steering committee. This approach led to the identification of issues and challenges associated with FAIRfying legacy data. In addition, it resulted in a deeper understanding of what is needed to improve the EDISON platform for processing clinical trial data. More details about the FAIRification of clinical trial data can be found described as a use case in the Pistoia Alliance Toolkit: FAIRification of clinical trial data
Corporate Linked Data (COLID) at Bayer
Bayer have developed and open sourced a platform named Corporate Linked Data (COLID). This is a technical solution designed for corporate environments that provides a metadata repository for corporate assets based upon semantic models. COLID assigns URIs as persistent and global unique identifiers to any resource. The incorporated network proxy ensures that these URIs are resolvable and can be used to directly access those assets. By following the Linked Data principles consequently, the data model of COLID uses RDF and provides the content through a SPARQL endpoint to consumers. This model was developed based on learnings from open standards like dcat and prov-o. Being both a management system for resolvable identifiers and an asset catalogue, COLID is the core service to realise Linked Data in corporate environments and therefore an essential cornerstone for implementation of FAIR data management. The documentation that describes COLID in comprehensive detail can be found on GitHub - which includes a quick start section.
Identifier policy at AstraZeneca
AstraZeneca has implemented a Uniform Resource Identifiers (URI) policy to construct a FAIR infrastructure across the global enterprise. This important corporate policy describes how URIs need to be constructed to facilitate cross-enterprise Findability, Interoperability and Reuse of digital objects. Significant adoption benefits occur in information domains where it is necessary to utilise data across multiple sources and where it is possible that there is no control over the information architecture within these sources. The business areas taking advantage of this approach include clinical studies, translational medicine and competitive intelligence. Further details about this identifier policy are detailed as a use case in the Pistoia Alliance Toolkit: Adoption and Impact of an Identifier Policy.
Education and training
Education and training are critically important for successful implementation of FAIR data management for clinical and non-clinical data, including the setting of the pharmaceutical and biotechnology enterprise. Educational and training material on FAIR data management is readily available in the scientific literature and as relevant web resources (examples).
An important aspect of education about FAIR implementation is understanding the process of data stewardship. This is a coordinated set of activities that ensure that data and associated metadata are made sufficiently FAIR in a sustainable manner. This means that FAIR data management is an ongoing and iterative process that is continually refined and tailored to suit the needs of a particular business application or use case as described in the Data stewardship handbook (HANDS). Although HANDS was written primarily for academic institutions, most aspects can be transferred to meet the needs of any industrial organisation. More details on the process of data stewardship can be found in the Pistoia Alliance FAIR Toolkit: Data Stewardship.
A highly effective approach for training on FAIR data management is the “Bring Your Own Data” (BYOD) or datathon workshop. These typically take place over two or three days so that participants can learn the practicalities of how to improve the FAIRness of their data and the benefits gained by making the data FAIR. It is a lightweight, very effective and enjoyable way to collaborate across scientific teams in multinational organisations. The BYOD or datathon method summarised here is based on that described in detail at the Dutch Techcentre for Life Sciences (DTL).
Data owners, domain experts (usually biologists or chemists), and FAIR data experts jointly work on specific data sets at a workshop. At the start, data owners present the data they wish to make FAIR. The data experts have extensive knowledge about FAIR data formats and principles, and support the data owners in choosing the optimal profile for making the data FAIR. In addition, they make sure that FAIR linked data is produced in the end. Domain experts can assist the data owners and data experts to solve intellectually challenging data modelling issues and to demonstrate the added value of FAIR data in answering specific research questions. More information on BYOD and datathon workshops can be found in the Pistoia Alliance FAIR Toolkit: BYOD Datathon Workshops.
Data-centric culture and the FAIR mindset
The trend emerging during recent years is to build a data-centric culture powered by FAIR data, which is seen by stakeholders as a valuable corporate asset. This contrasts with the traditional application-centric approach, to regard data as a secondary commodity. This contributes to limited reusability of valuable clinical data, typically buried in data silos or graveyards.
The introduction section of this guide has described how implementation of the FAIR principles results in realisation of much more value from clinical data over a much longer period because it is much more likely to be reusable (see figure 1). This shift in recognition and realisation of much greater value for data and metadata at the centre of a business organisation, defines a data-centric culture.
To support this seismic shift to a data-centric culture, the most progressive and successful pharmaceutical and biotechnology companies now recognise the importance of FAIR implementation as a change in data management culture for data. This journey is long and arduous and thus can be best achieved in an iterative manner.
However, it must be said that although making data FAIR is important, this is not an end in itself, but rather a powerful enabler. It supports digital transformation to increase business productivity and it is vital for success with data-hungry technologies such as machine learning and artificial intelligence.
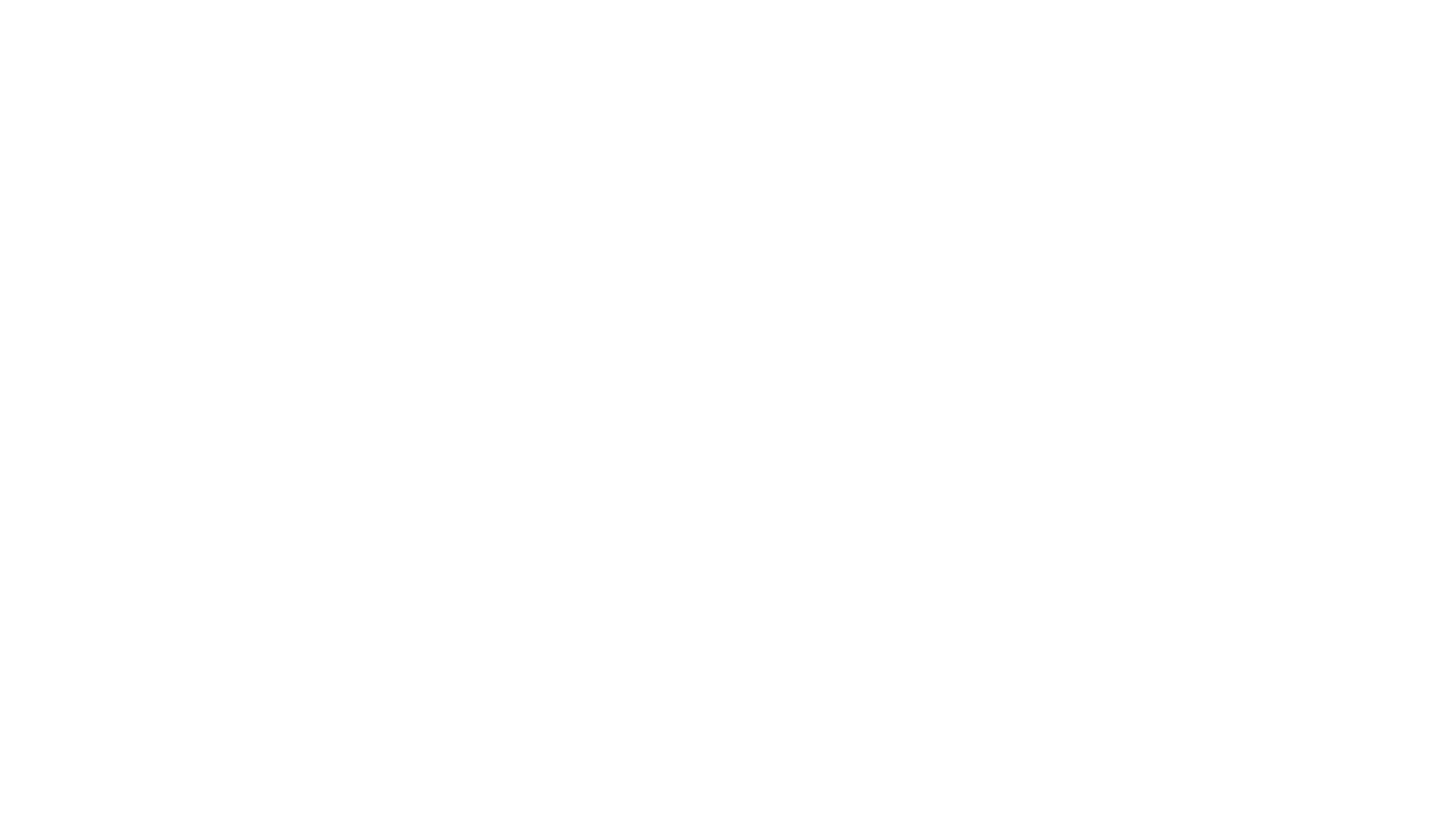